Introduction
Artificial intelligence (AI) was once a concept of the future but is now a reality that continues to transform the way businesses work in today's fast-paced tech world. As AI continues to extend its reach into various industries, the demand for robust IT infrastructure capable of training AI, and handling AI workloads is skyrocketing.
More money is being poured into research, and companies are leveraging foundational AI models to build custom solutions for their workflow. But here's the million-dollar question: Is your IT infrastructure ready for the AI revolution?
Let’s paint the picture to illustrate the impact of artificial intelligence. According to Grand View Research, the global artificial intelligence market size is expected to reach $1,811.75 billion by 2030. This expected explosive growth represents a shift in how businesses will operate, compete, and innovate in the coming years.
As AI becomes more and more intertwined in every industry, it places unprecedented demands on the computing infrastructure that power these complex models. From processing vast amounts of data to running complex algorithms in real-time, AI workloads represent a distinct category, fundamentally different from conventional computing tasks. This is why preparing your IT infrastructure for future AI demands isn't just a good idea – it's a necessity for staying competitive in the AI-driven future.
Infrastructure Challenges in AI Workloads
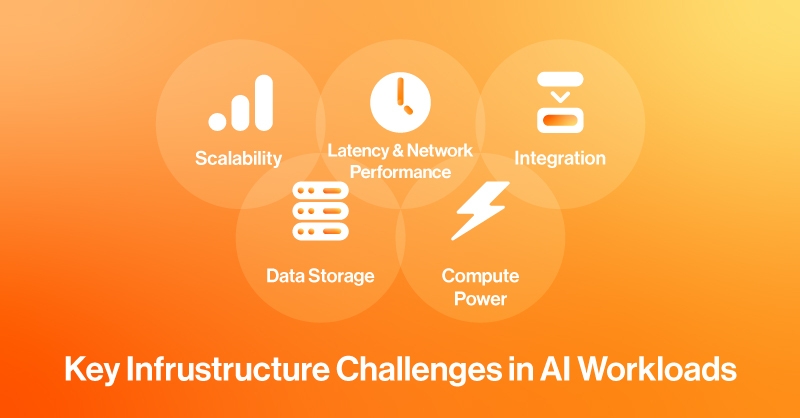
Scalability
AI workloads are notoriously unpredictable from project to project. Advancements in an existing AI model to increase fidelity via parameter tuning or additional data points require additional computing performance. One moment, the system may be operating smoothly, and the next version could be hit with a massive spike in demand.
Furthermore, exploratory data analysis and the development of other models can saturate resources and affect day-to-day operations. The landscape of AI models and testing new methodologies allows for business flexibility and innovation required to stay competitive. This unpredictability means that relying on rigid, unchanging infrastructure is insufficient.
The solution? Elastic infrastructure that can scale up or down based on demand. Cloud computing platforms can supplement spikes in demand. Continued expansion in your computing infrastructure and dedicated hardware to daily operations and innovation can separate workloads. Ensuring you have enough computing to spare can work wonders for AI advancements research continues on the backend.
Data Storage
AI demands substantial quantities of data. Machine learning, deep learning, data science, and even just simple data analysis algorithms require massive amounts of data for training and inference.
According to IDC, the global datasphere is projected to grow from 33 Zettabytes in 2018 to 175 Zettabytes by 2025. A significant portion of this growth will be driven by AI and IoT devices.
To handle this data deluge, organizations need high-performance, scalable storage solutions. Technologies like software-defined storage (SDS) and object storage are becoming increasingly popular for AI workloads due to their scalability and ability to handle unstructured data efficiently.
We also recommend the adoption of high-performance NVMe storage servers to enable high-speed and dense storage. This does come at a higher cost per TB. Time is money, thus the expensive yet fast and dense storage is a necessary tradeoff for certain AI workloads.
Latency and Network Performance
Many AI applications, particularly those involving real-time decision-making or edge computing, are extremely sensitive to latency. Even a few milliseconds of delay can make a significant difference in applications like autonomous vehicles or high-frequency trading.
To address this challenge, ensure your data center or computing infrastructure is capable or has installed high-speed networking technologies like InfiniBand and 100 Gigabit Ethernet. Additionally, deploying edge computing hardware can bring the computation closer to the data source, reducing latency.
Compute Power
If data is the fuel of AI, then compute power is its engine. AI workloads, especially during the training phase of deep learning models, require massive amounts of computational resources. Traditional CPUs-only clusters don’t cut it anymore due to the lack of parallelism built into the processor. While still important to the system as a whole, GPUs are the standout choice for effective and competitive AI research.
NVIDIA is the leader in this space as the main source of B2B and B2C suppliers of high-performance GPUs suitable for AI. Their integration with CUDA and AI frameworks is an invaluable advantage; its existing adoption and familiarity of the CUDA framework in other productivity applications have in-turn, enabled NVIDIA to be a leader in the accelerated hardware space and critical for specialized computing AI infrastructures.
We recommend NVIDIA Grace and NVIDIA HGX solutions to those looking to expand or build out their computing infrastructure. Or configure a server supporting up to 8x GPUs in a familiar PCIe form factor.
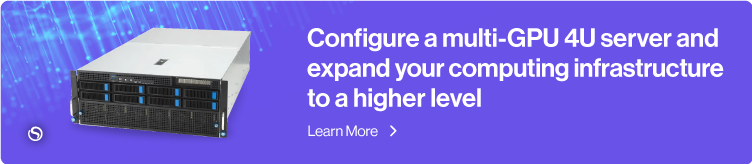
Compliance
As Artificial Intelligence models become more prevalent, so do the regulations. Increasingly sensitive data and critical decisions, security, and compliance become paramount to maintain a safe and effective business approach to not work backward:
- Data Privacy: Implement robust data encryption and access control mechanisms. Be aware of regulations like GDPR and CCPA that govern data usage in AI systems. If there are data leaks, significant backlash can cause business disruption.
- Explainability and Transparency: As AI systems make more decisions, ensure you can explain how these decisions are made. This is crucial for both regulatory compliance and building trust with users.
- Bias and Fairness: Implement processes to detect and mitigate bias in AI models. This is not just an ethical consideration but also a growing regulatory concern.
Key Takeaways: Preparing for the AI Future
As we wrap up, let's recap the key strategies for future-proofing your IT infrastructure for AI workloads:
- Embrace hybrid cloud deployments to pair your on-prem for flexibility and scalability.
- Invest in high-performance, scalable data storage solutions.
- Optimize your networking for high bandwidth and low latency.
- Leverage GPUs and specialized hardware for AI computations and accelerated computing.
- Implement automation and orchestration tools for efficient management.
- Ensure seamless integration between new AI systems and existing infrastructure. You can achieve this by choosing a solutions integrator that resonates with you.
- Prioritize security and compliance in your AI infrastructure.
- Build for long-term maintainability and upgradability.
The AI revolution is here, and it's transforming the business landscape at an unprecedented pace. By future-proofing your IT infrastructure now, you're not just preparing for the future – you're positioning your organization to lead in the AI-driven world of tomorrow.
Remember, the goal isn't to predict the future with perfect accuracy but to build infrastructure flexible enough to adapt to whatever the future may bring. So start planning, start investing, and contact us to start building. Contact SabrePC to get started today; we consult according your unique workload, configure an ideal solution, and deliver turnkey systems ready to deploy upon delivery and built to scale towards the future.